Publications Neuro-inspired Theory, Modeling and Applications

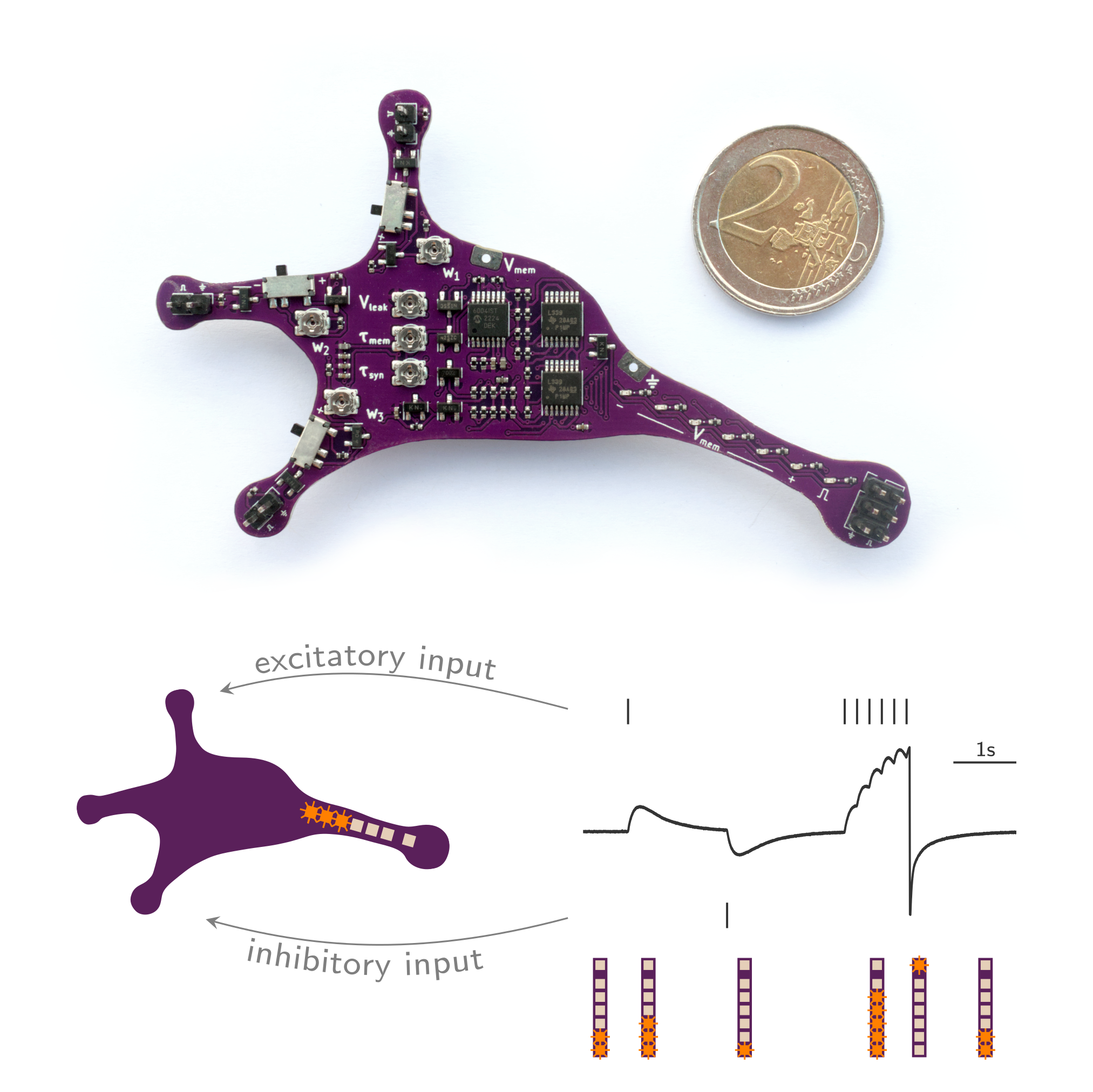
Y. Stradmann, J. Göltz, M.A. Petrovici, J. Schemmel, S. Billaudelle.
Lu.i – A low-cost electronic neuron for education and outreach. Trends in Neuroscience and Education 2025 DOI pdf
Lu.i – A low-cost electronic neuron for education and outreach. Trends in Neuroscience and Education 2025 DOI pdf
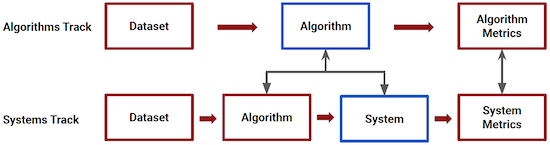
J. Yik, ..., M.A. Petrovici et al..
NeuroBench: Advancing Neuromorphic Computing through Collaborative, Fair and Representative Benchmarking. Nature Communications 2025 DOI pdf
NeuroBench: Advancing Neuromorphic Computing through Collaborative, Fair and Representative Benchmarking. Nature Communications 2025 DOI pdf
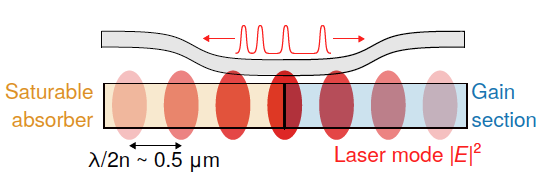

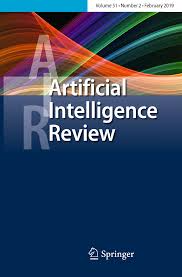
M. Farisco, G. Baldassarre, E. Cartoni, A. Leach, M.A. Petrovici, A. Rosemann, A. Salles, B. Stahl, S. J. van Albada.
A method for the ethical analysis of brain-inspired AI. Artificial Intelligence Review 2024 DOI pdf
A method for the ethical analysis of brain-inspired AI. Artificial Intelligence Review 2024 DOI pdf
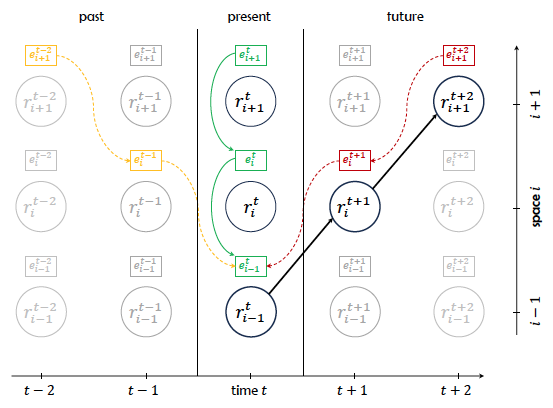
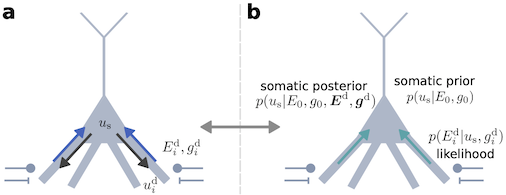
J. Jordan, J. Sacramento, W.A.M. Wybo, M.A. Petrovici, W. Senn.
Conductance-based dendrites perform Bayes-optimal cue integration. PLoS Comput Biol 2024 DOI pdf
Conductance-based dendrites perform Bayes-optimal cue integration. PLoS Comput Biol 2024 DOI pdf
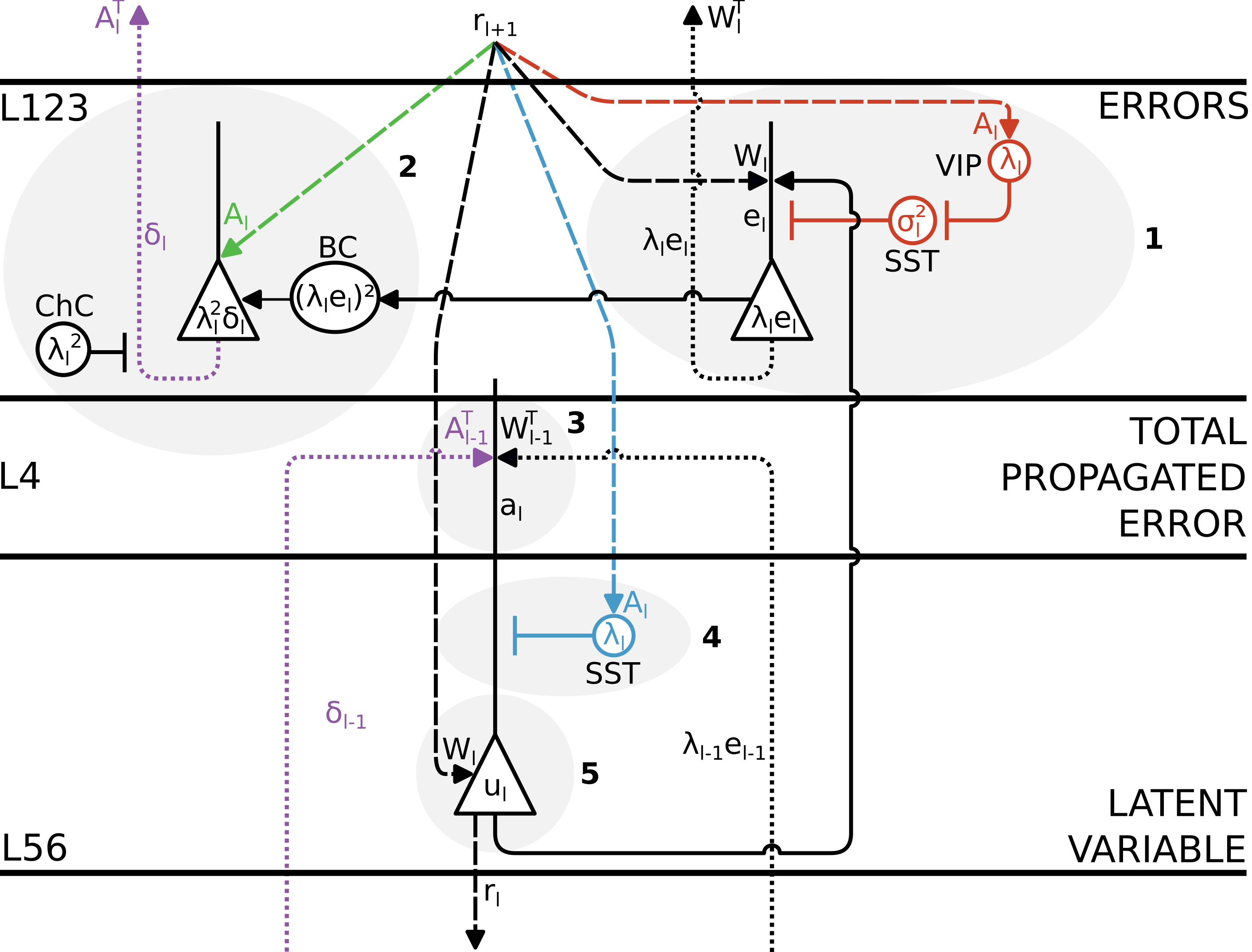
A. Granier, M.A. Petrovici, W. Senn, K.A. Wilmes.
Confidence and second-order errors in cortical circuits. PNAS Nexus 2024 DOI pdf
Confidence and second-order errors in cortical circuits. PNAS Nexus 2024 DOI pdf

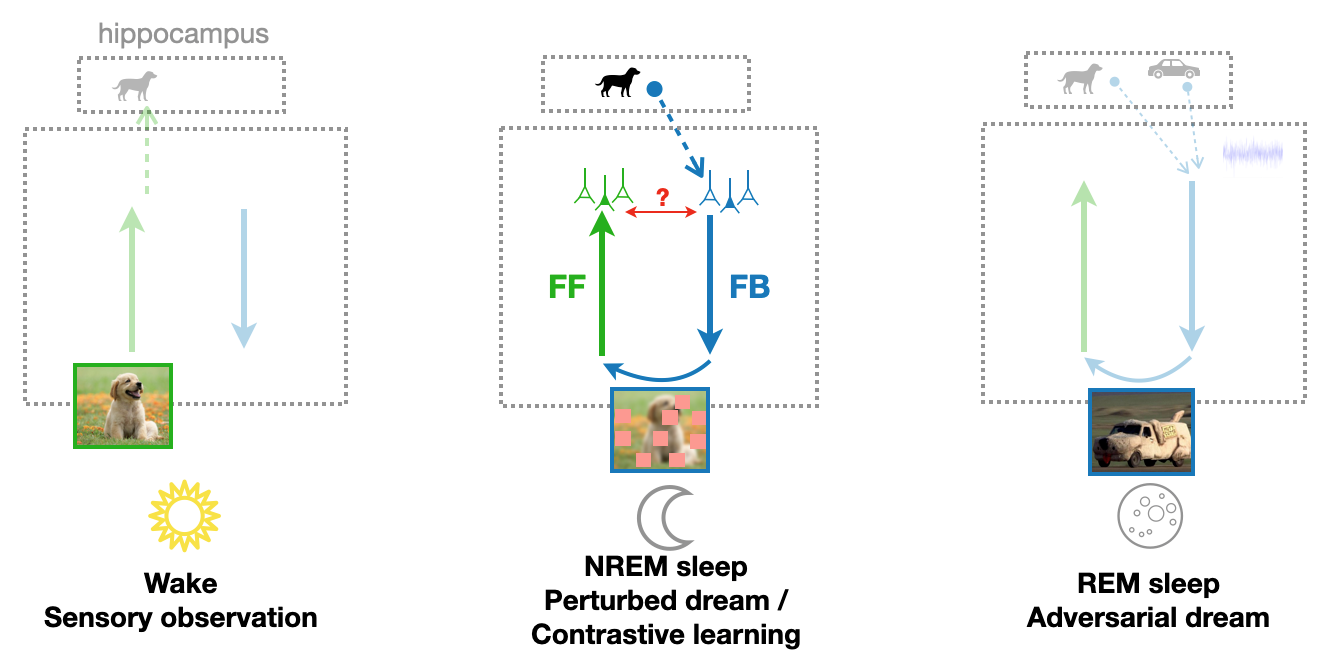
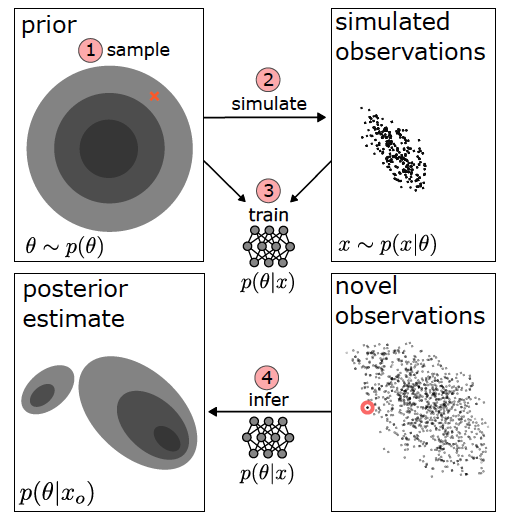
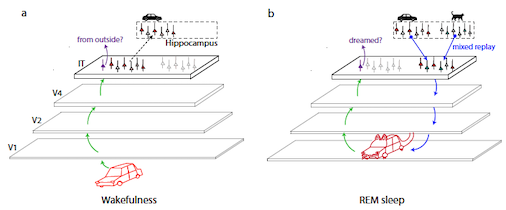
N. Deperrois, M.A. Petrovici, W. Senn, J. Jordan.
Learning beyond sensations: how dreams organize neuronal representations. Neuroscience & Biobehavioral Reviews 2024 DOI pdf
Learning beyond sensations: how dreams organize neuronal representations. Neuroscience & Biobehavioral Reviews 2024 DOI pdf
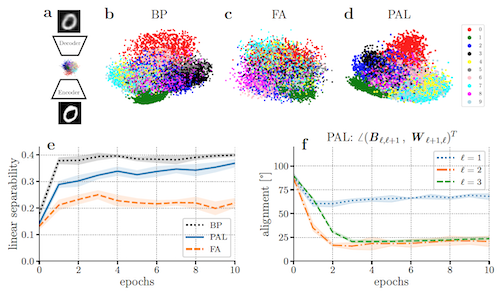
K. Max, L. Kriener, G. Pineda Garcia, T. Nowotny, I. Jaras, W. Senn and M.A. Petrovici.
Learning efficient backprojections across cortical hierarchies in real time. Nature Machine Intelligence 6, 619–630, 2024 DOI pdf
Learning efficient backprojections across cortical hierarchies in real time. Nature Machine Intelligence 6, 619–630, 2024 DOI pdf
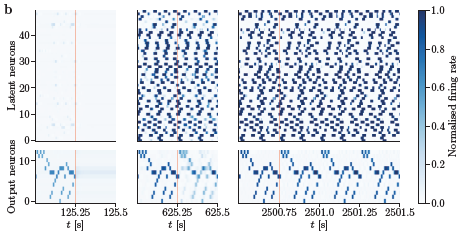
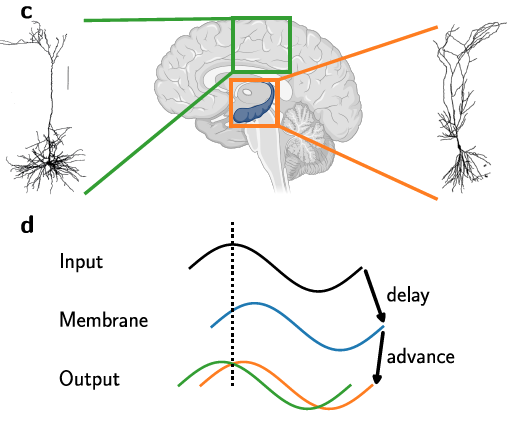
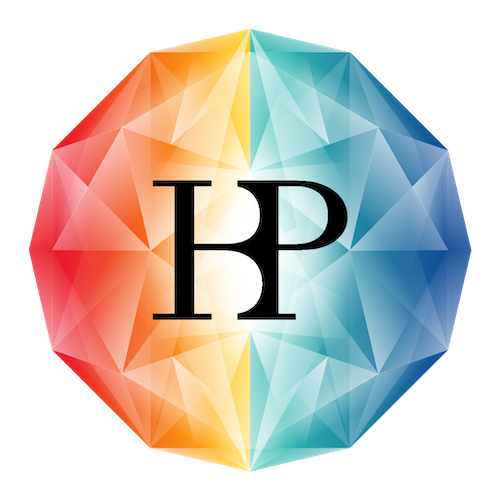
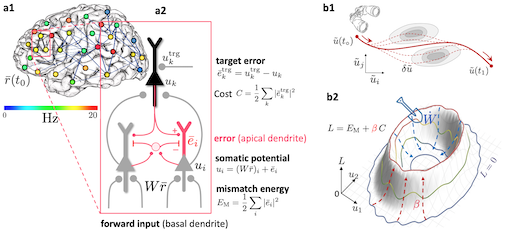
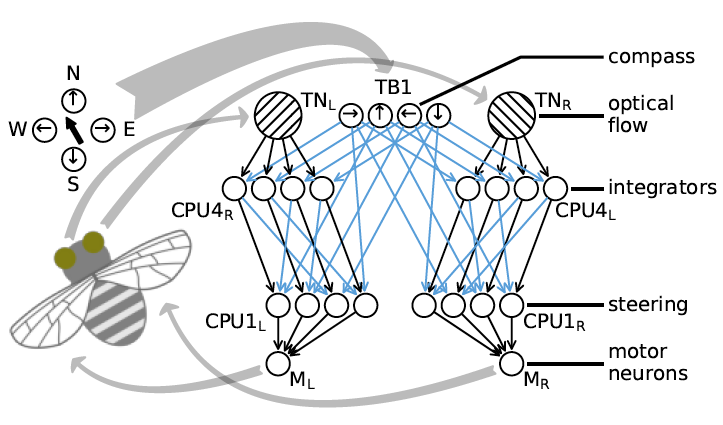
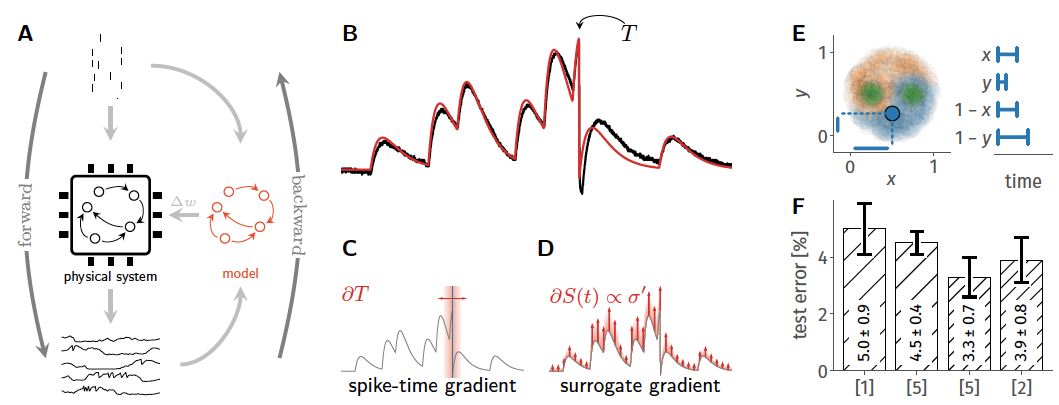
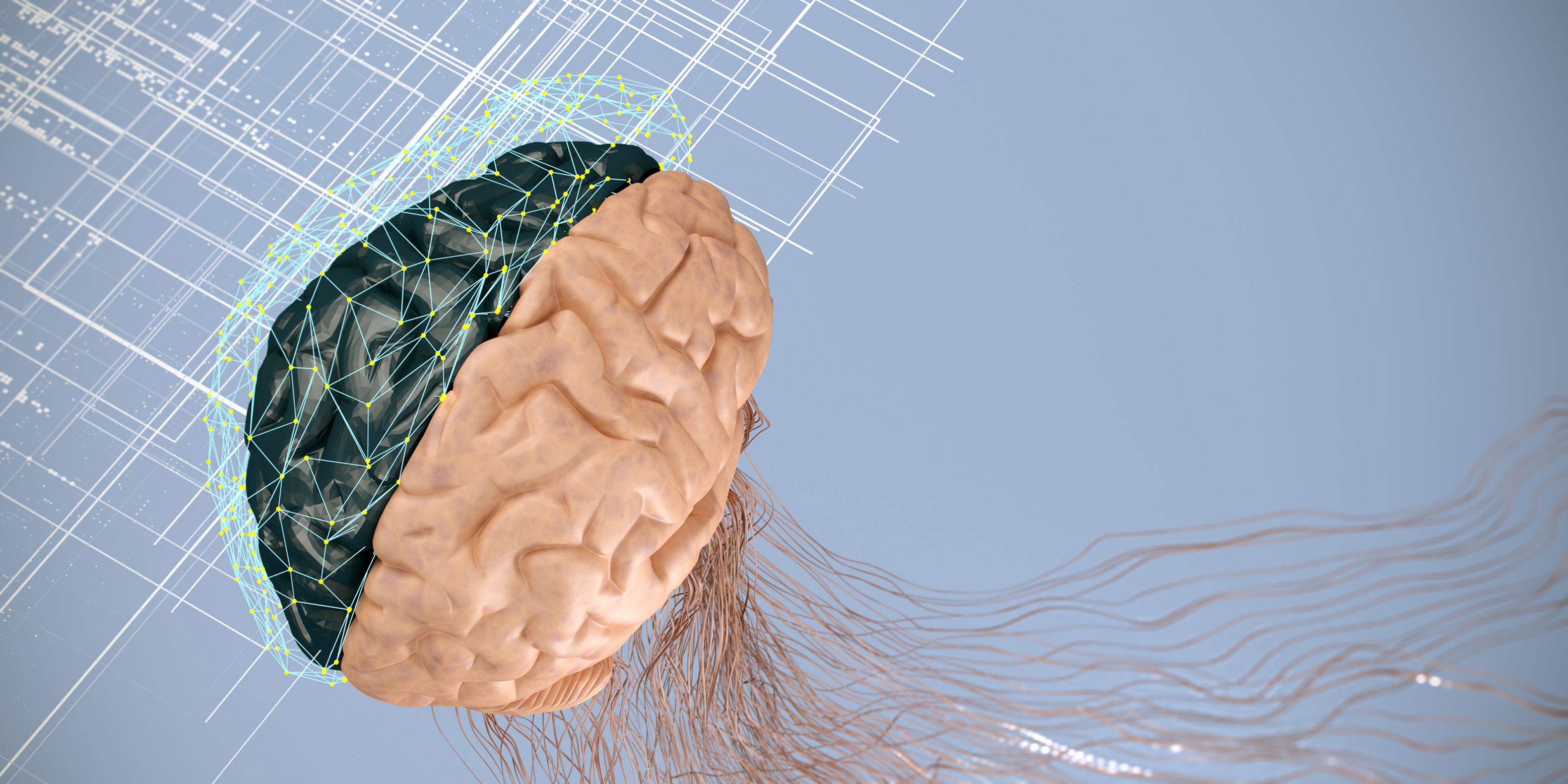
S. Chakrabarty, M.A. Petrovici.
Is neuromorphic computing disruptive enough to 1) advance our understanding of the brain and 2) make the design and working of (bio)electronic devices efficient and scalable?. Research Directions: Bioelectronics 2023 DOI pdf
Is neuromorphic computing disruptive enough to 1) advance our understanding of the brain and 2) make the design and working of (bio)electronic devices efficient and scalable?. Research Directions: Bioelectronics 2023 DOI pdf
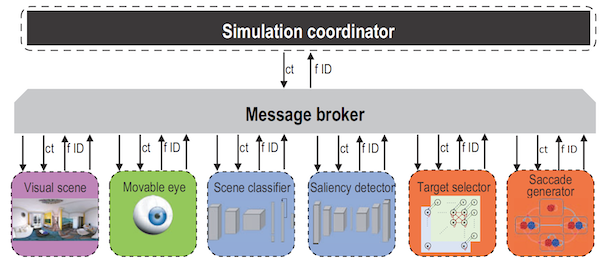
M. Senden, S.J. van Albada, G. Pezzulo, E. Falotico, I. Hashim, A. Kroner, A.C. Kurth, P. Lanillos, V. Narayanan, C. Pennartz, M.A. Petrovici, L. Steffen, T. Weidler, R. Goebel.
Modular-integrative modeling: a new framework for building brain models that blend biological realism and functional performance. National Science Review 2023 DOI pdf
Modular-integrative modeling: a new framework for building brain models that blend biological realism and functional performance. National Science Review 2023 DOI pdf
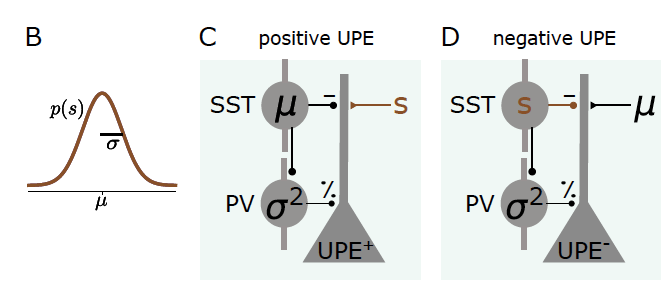
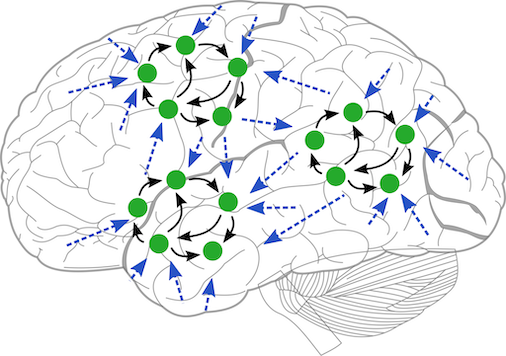
A. Korcsak-Gorzo, M.G. Müller, A. Baumbach, L. Leng, O.J. Breitwieser, S.J. van Albada, W. Senn, K. Meier, R. Legenstein, M. A. Petrovici.
Cortical oscillations support sampling-based computations in spiking neural networks. PLoS Comput Biol 2022 DOI PubMed pdf
Cortical oscillations support sampling-based computations in spiking neural networks. PLoS Comput Biol 2022 DOI PubMed pdf
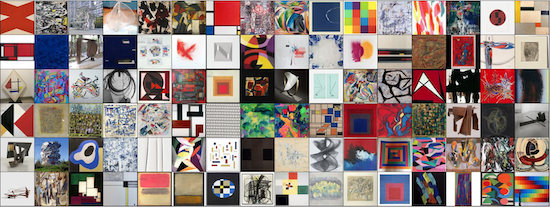
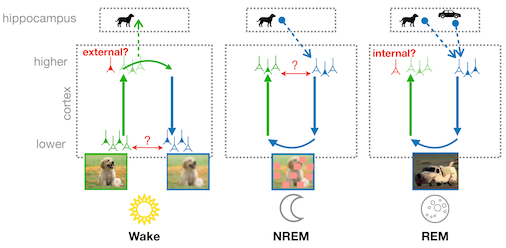
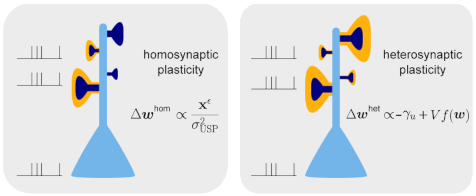
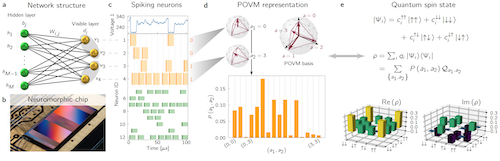
S. Czischek, A. Baumbach, S. Billaudelle, B. Cramer, L. Kades, J.M. Pawlowski, M.K. Oberthaler, J. Schemmel, M.A. Petrovici, T. Gasenzer and M. Gärttner.
Spiking neuromorphic chip learns entangled quantum states. SciPost Physics 39, 2022 DOI pdf
Spiking neuromorphic chip learns entangled quantum states. SciPost Physics 39, 2022 DOI pdf
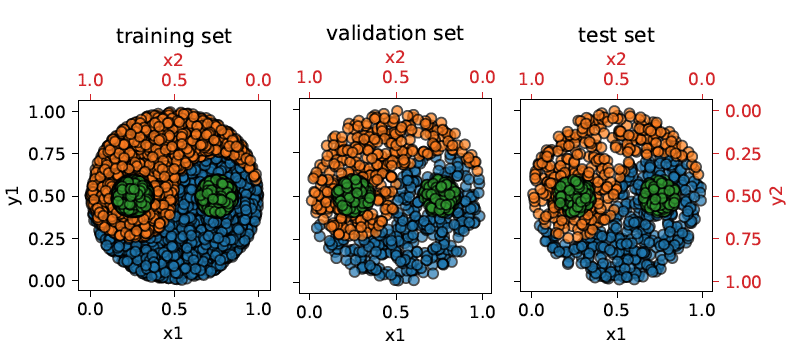
L. Kriener, J. Göltz, M. A. Petrovici.
The Yin-Yang dataset. Neuro-Inspired Computational Elements Conference Proceedings 2022 DOI pdf
The Yin-Yang dataset. Neuro-Inspired Computational Elements Conference Proceedings 2022 DOI pdf
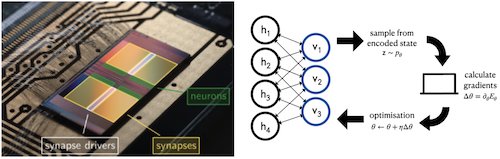
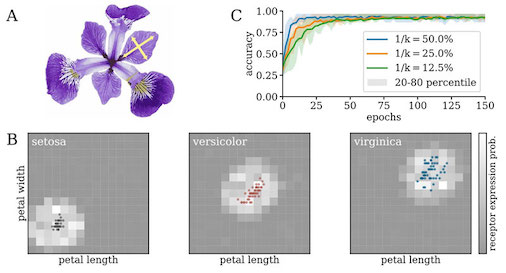
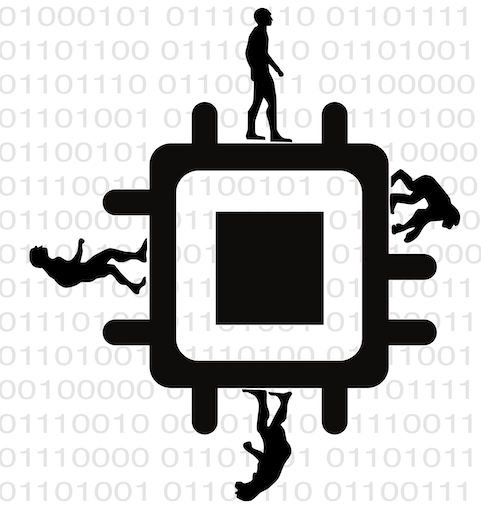
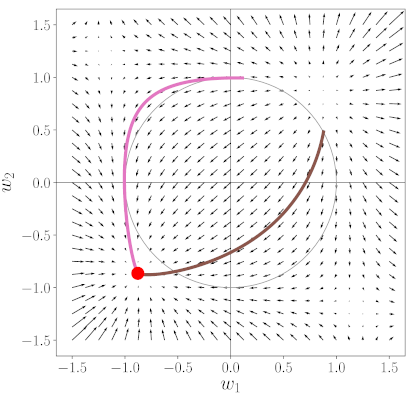
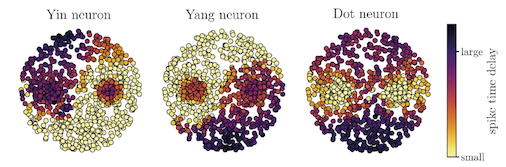
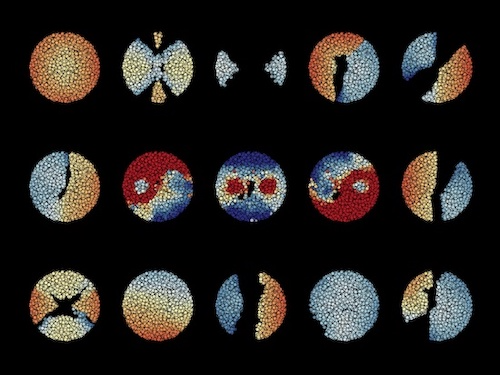
J. Göltz, L. Kriener, A. Baumbach, S. Billaudelle, O. Breitwieser, B. Cramer, D. Dold, A.F. Kungl, W. Senn, J. Schemmel, K. Meier, M.A. Petrovici.
Fast and energy-efficient neuromorphic deep learning with first-spike times. Nature Machine Intelligence 823–835, 2021 DOI pdf
Fast and energy-efficient neuromorphic deep learning with first-spike times. Nature Machine Intelligence 823–835, 2021 DOI pdf
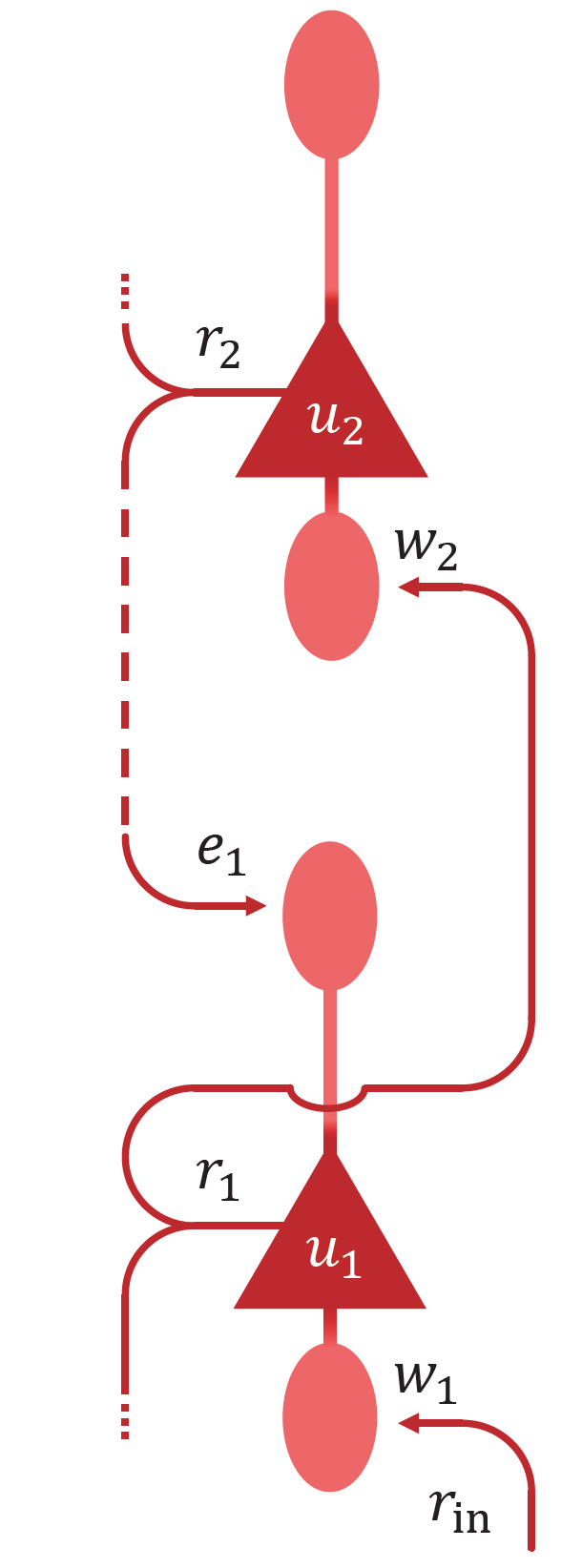
P. Haider, B. Ellenberger, L. Kriener, J. Jordan, W. Senn, M.A. Petrovici.
Latent Equilibrium: A unified learning theory for arbitrarily fast computation with arbitrarily slow neurons. Advances in Neural Information Processing Systems (NeurIPS) 2021 pdf
Latent Equilibrium: A unified learning theory for arbitrarily fast computation with arbitrarily slow neurons. Advances in Neural Information Processing Systems (NeurIPS) 2021 pdf
Peer-reviewed conference article - Advances in Neural Information Processing Systems 34 (NeurIPS 2021)
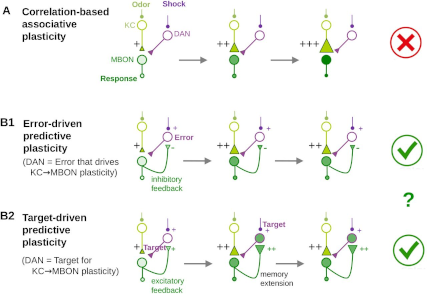
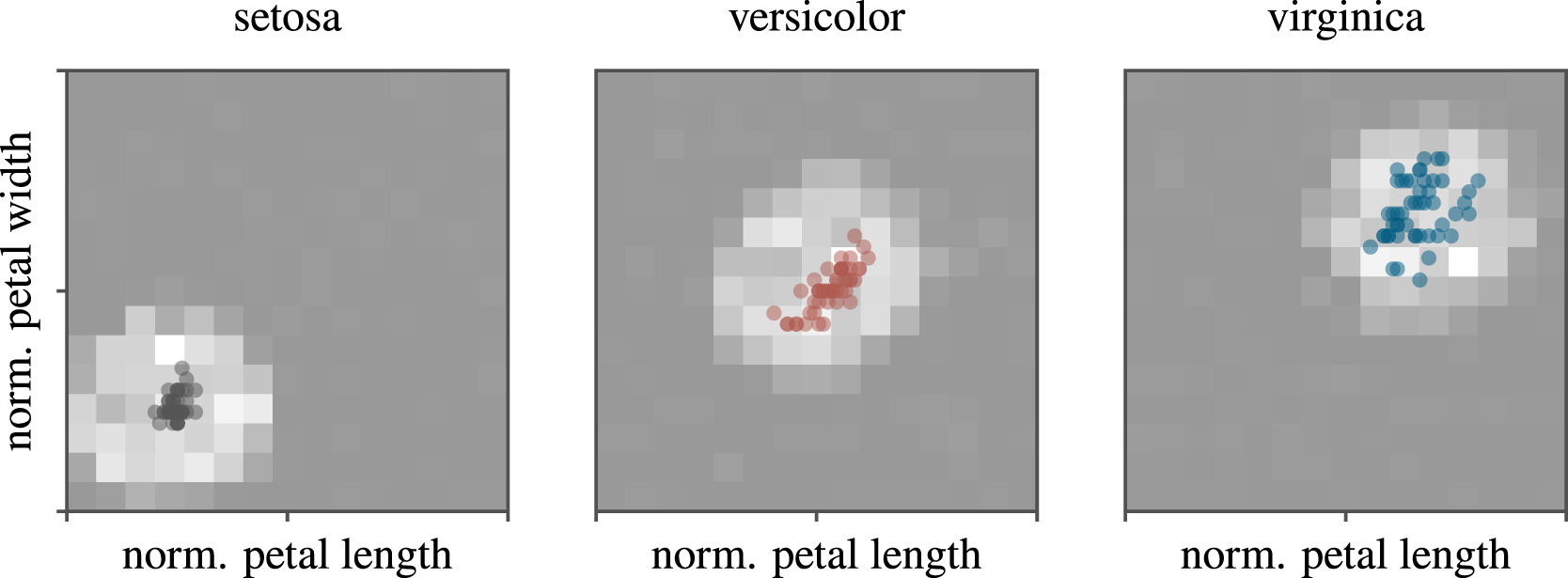
S. Billaudelle, B. Cramer, M.A. Petrovici, K. Schreiber, D. Kappel, J. Schemmel, K. Meier.
Structural plasticity on an accelerated analog neuromorphic hardware system. Neural Networks 133, 11-20, 2021 DOI pdf
Structural plasticity on an accelerated analog neuromorphic hardware system. Neural Networks 133, 11-20, 2021 DOI pdf
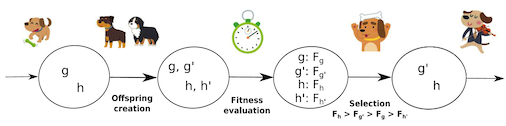
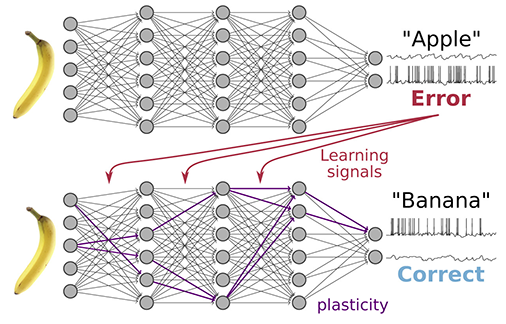
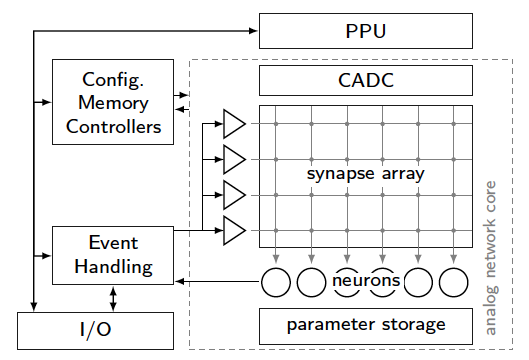
S. Billaudelle, Y. Stradmann, K. Schreiber, B. Cramer, A. Baumbach, D. Dold, J. Göltz, A.F. Kungl, T.C. Wunderlich, A. Hartel, E. Müller, O. Breitwieser, C. Mauch, M. Kleider, A. Grübl, Da. Stöckel, C. Pehle, A. Heimbrecht, P. Spilger, G. Kiene, V. Karasenko, W. Senn, M.A. Petrovici, J. Schemmel, K. Meier.
Versatile emulation of spiking neural networks on an accelerated neuromorphic substrate. IEEE Xplore 2020 DOI pdf
Versatile emulation of spiking neural networks on an accelerated neuromorphic substrate. IEEE Xplore 2020 DOI pdf
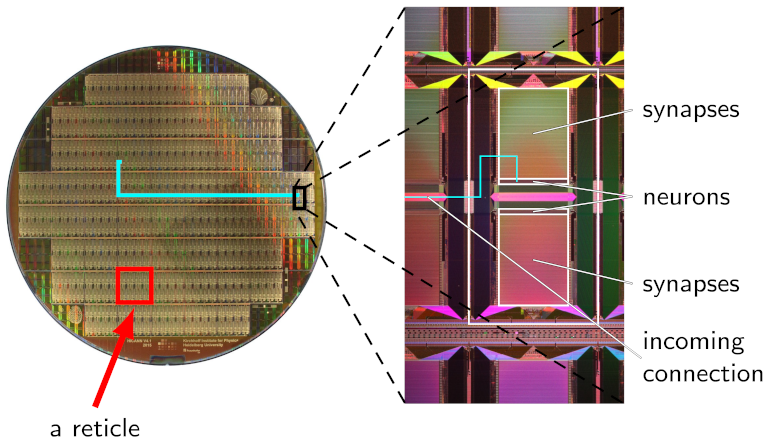
A.F. Kungl, S. Schmitt, J. Klähn, P. Müller, A. Baumbach, D. Dold, A. Kugele, N. Gürtler, E. Müller, C. Koke, M. Kleider, C. Mauch, O. Bretwieser, M. Güttler, D. Husmann, K. Husmann, J. Ilmberger, A. Hartel, V. Karasenko, A. Grübl, J. Schemmel, K. Meier, M.A. Petrovici.
Accelerated physical emulation of Bayesian inference in spiking neural networks. Front. Neuroscience 2019 DOI pdf
Accelerated physical emulation of Bayesian inference in spiking neural networks. Front. Neuroscience 2019 DOI pdf
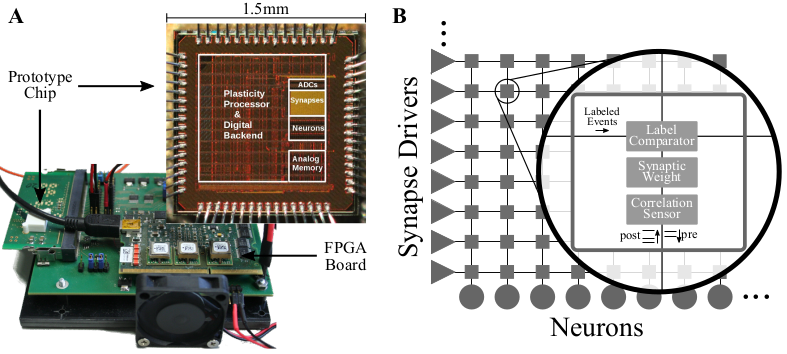
T. Wunderlich, A.F. Kungl, E. Müller, A. Hartel, Y. Stradmann, S. A. Aamir, A. Grübl, A. Heimbrecht, K. Schreiber, D. Stöckel, C. Pehle, S. Billaudelle, G. Kiene, C. Mauch, J. Schemmel, K. Meier and M.A. Petrovici.
Demonstrating Advantages of Neuromorphic Computation: A Pilot Study. Front. Neuroscience 3:260, 2019 DOI pdf
Demonstrating Advantages of Neuromorphic Computation: A Pilot Study. Front. Neuroscience 3:260, 2019 DOI pdf
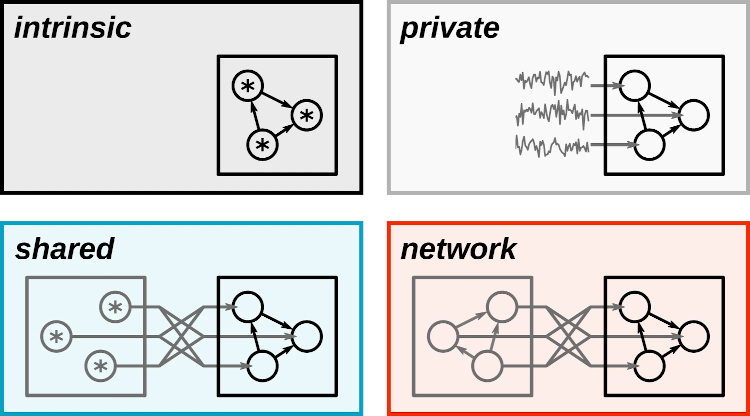
J. Jordan, M.A. Petrovici, O. Breitwieser, J. Schemmel, K. Meier, M. Diesmann & T. Tetzlaff.
Deterministic networks for probabilistic computing. Nature Scientific Reports 9:18303, 2019 DOI pdf
Deterministic networks for probabilistic computing. Nature Scientific Reports 9:18303, 2019 DOI pdf
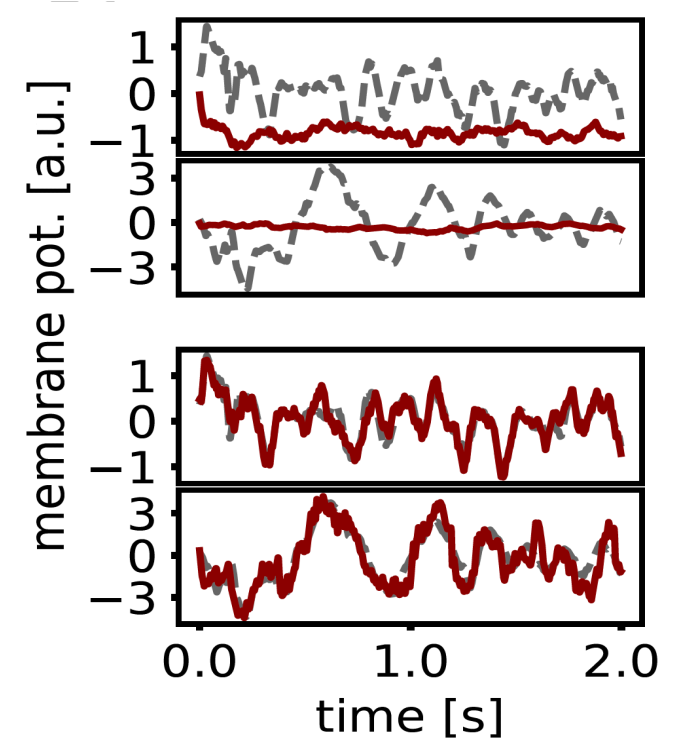
D. Dold, A.F. Kungl, J. Sacramento, M.A. Petrovici, K. Schindler, J. Binas, Y. Bengio, W. Senn.
Lagrangian neurodynamics for real-time error-backpropagation across cortical areas. 2019 pdf
Lagrangian neurodynamics for real-time error-backpropagation across cortical areas. 2019 pdf
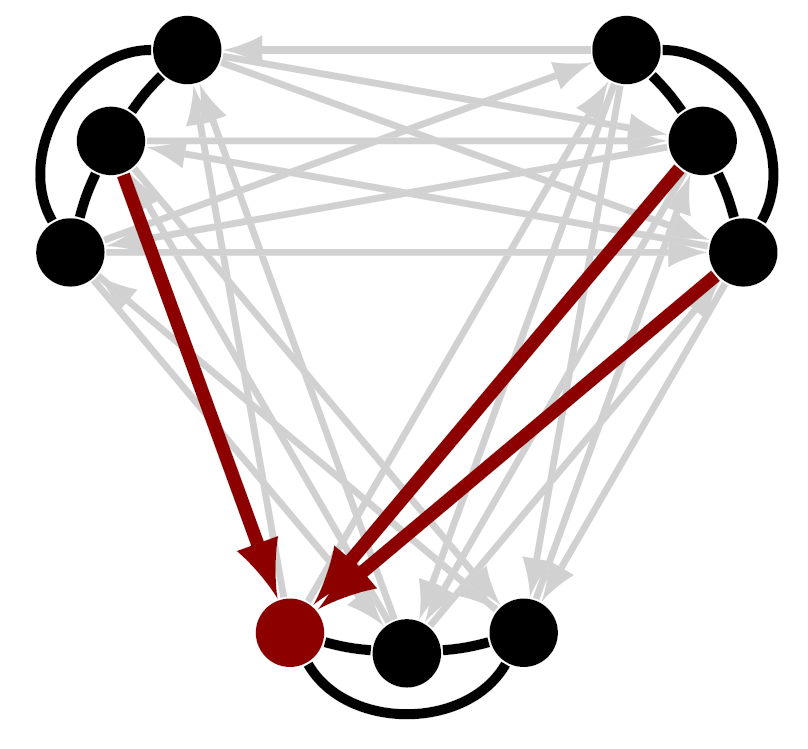
D. Dold, I. Bytschok, A.F. Kungl, A. Baumbach, O. Breitwieser, W. Senn, J. Schemmel, K. Meier, M.A. Petrovici.
Stochasticity from function - Why the Bayesian brain may need no noise. Neural Networks 2019 DOI pdf
Stochasticity from function - Why the Bayesian brain may need no noise. Neural Networks 2019 DOI pdf
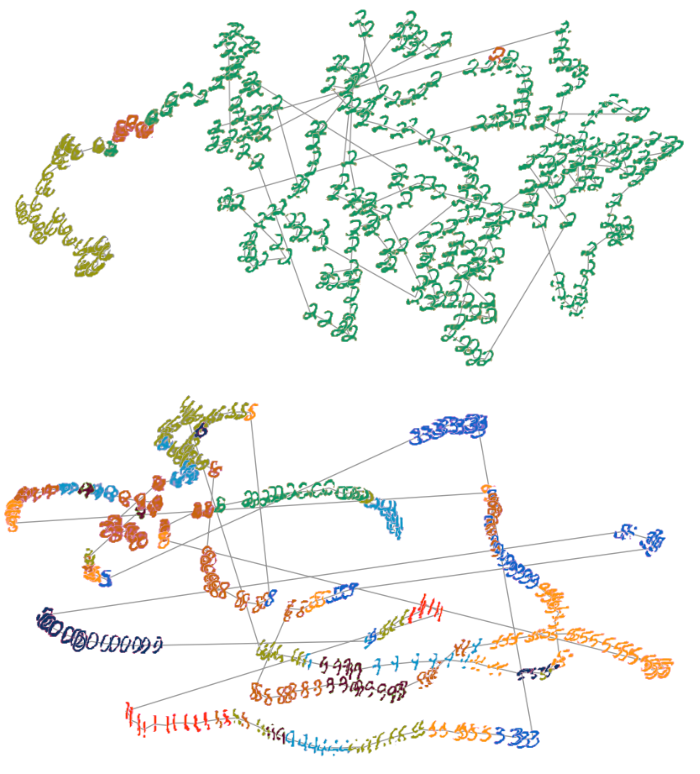
Luziwei Leng, R. Martel, O. Breitwieser, I. Bytschok, W. Senn, J. Schemmel, K. Meier & M.A. Petrovici .
Spiking neurons with short-term synaptic plasticity form superior generative networks. Nature Scientific Reports 8: 10651, 2018 DOI pdf
Spiking neurons with short-term synaptic plasticity form superior generative networks. Nature Scientific Reports 8: 10651, 2018 DOI pdf
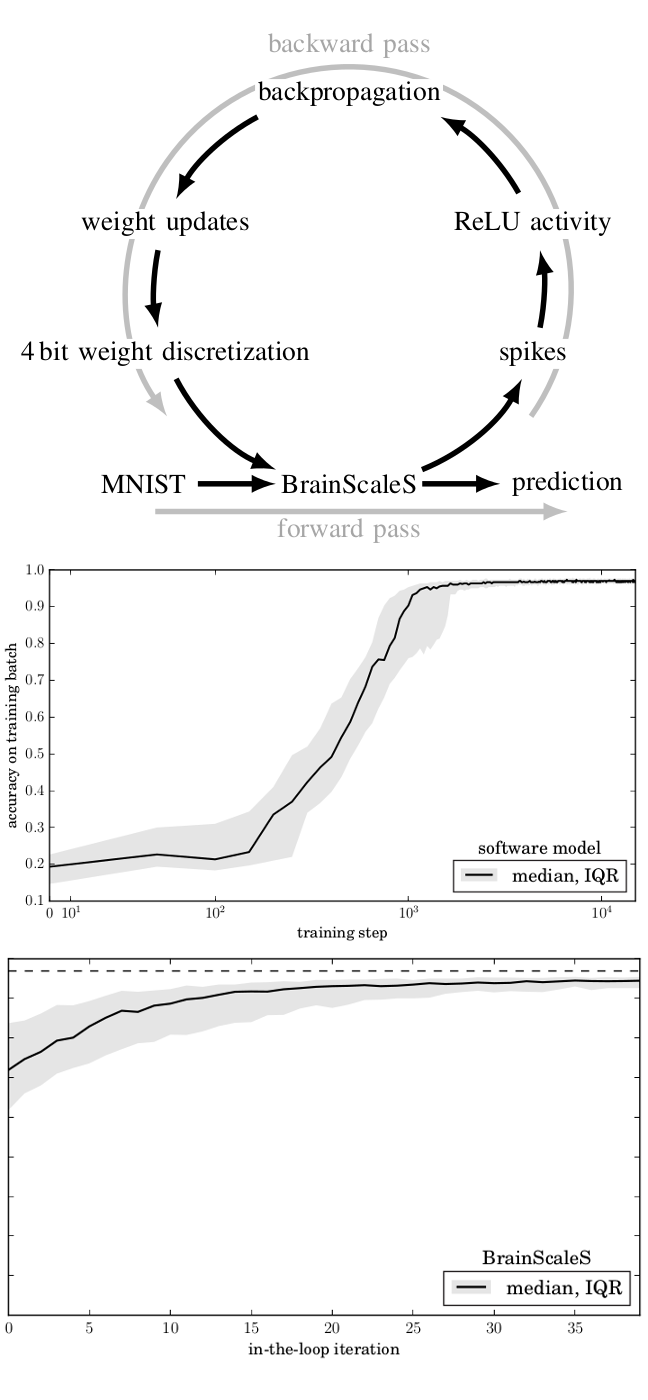
S. Schmitt, J. Klähn, G. Bellec, A. Grübl, M. Güttler, A. Hartel, S. Hartmann, D. Husmann, K. Husmann, S. Jeltsch, V. Karasenko, M. Kleider, C. Koke, A. Kononov, C. Mauch, E. Müller, P. Müller, J. Partzsch, M.A. Petrovici, S. Schiefer, S. Scholze, V. Thanasoulis, B. Vogginger, R. Legenstein, W. Maass, C. Mayr, R. Schüffny, J. Schemmel, K. Meier.
Neuromorphic hardware in the loop: Training a deep spiking network on the BrainScaleS wafer-scale system. Proceedings of 2017 IJCNN online, 2017 DOI pdf
Neuromorphic hardware in the loop: Training a deep spiking network on the BrainScaleS wafer-scale system. Proceedings of 2017 IJCNN online, 2017 DOI pdf
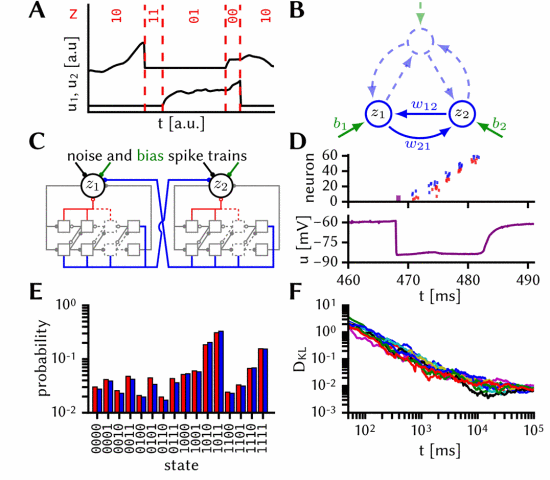
M.A. Petrovici, S. Schmitt, J. Klähn, D. Stöckel, A. Schroeder, G. Bellec, J. Bill, O. Breitwieser, I. Bytschok, A. Grübl, M. Güttler, A. Hartel, S. Hartmann, D. Husmann, K. Husmann, S. Jeltsch, V. Karasenko, M. Kleider, C. Koke, A. Kononov, C. Mauch, E. Müller, P. Müller, J. Partzsch, T. Pfeil, S. Schiefer, S. Scholze, A. Subramoney, V. Thanasoulis, B. Vogginger, R. Legenstein, W. Maass, R. Schüffny, C. Mayr, J. Schemmel, K. Meier.
Pattern representation and recognition with accelerated analog neuromorphic systems. Proceedings of 2017 IEEE International Symposium on Circuits and Systems (ISCAS) 2017 DOI pdf
Pattern representation and recognition with accelerated analog neuromorphic systems. Proceedings of 2017 IEEE International Symposium on Circuits and Systems (ISCAS) 2017 DOI pdf
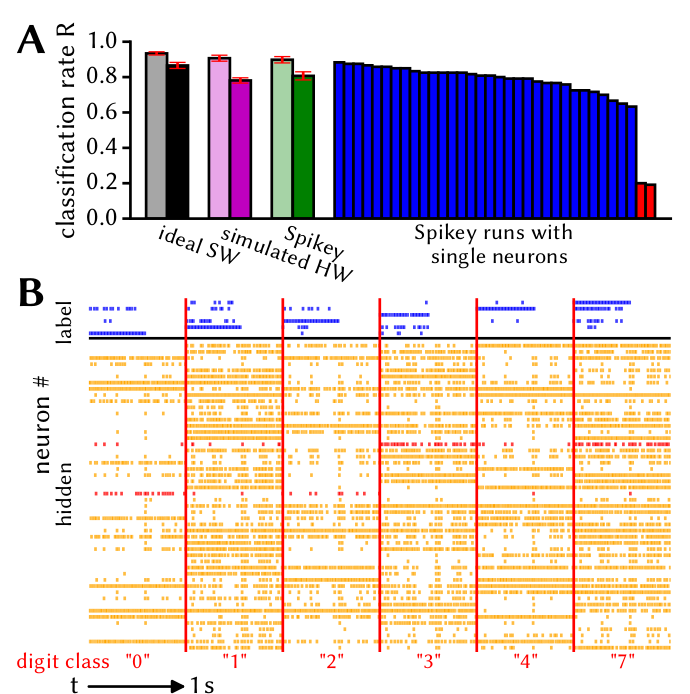
M.A. Petrovici, A. Schroeder, O. Breitwieser, A. Grübl, J. Schemmel, K. Meier.
Robustness from structure: Inference with hierarchical spiking networks on analog neuromorphic hardware. Proceedings of 2017 IJCNN online, 2017 DOI pdf
Robustness from structure: Inference with hierarchical spiking networks on analog neuromorphic hardware. Proceedings of 2017 IJCNN online, 2017 DOI pdf
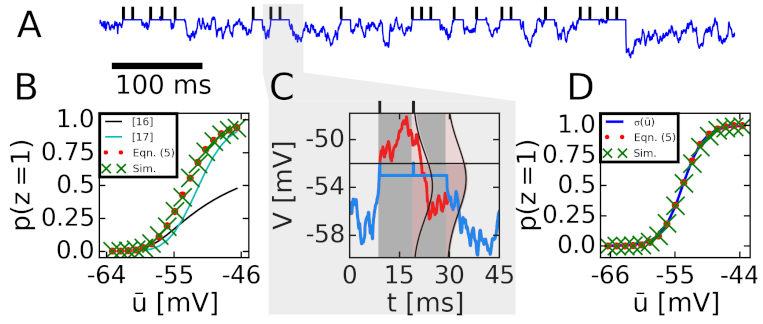
M.A. Petrovici, J. Bill, I. Bytschok, J. Schemmel, K. Meier.
Stochastic inference with spiking neurons in the high-conductance state. Phys. Rev. E 042312, 2016 DOI
Stochastic inference with spiking neurons in the high-conductance state. Phys. Rev. E 042312, 2016 DOI
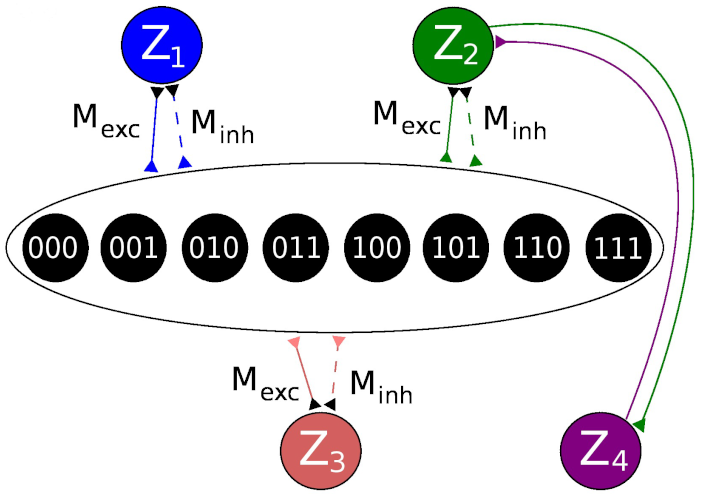
D. Probst, M.A. Petrovici, I. Bytschok, J. Bill, D. Pecevski, J. Schemmel, K. Meier.
Probabilistic inference in discrete spaces can be implemented into networks of LIF neurons. Front. Comput.Neurosci. 2015 DOI
Probabilistic inference in discrete spaces can be implemented into networks of LIF neurons. Front. Comput.Neurosci. 2015 DOI
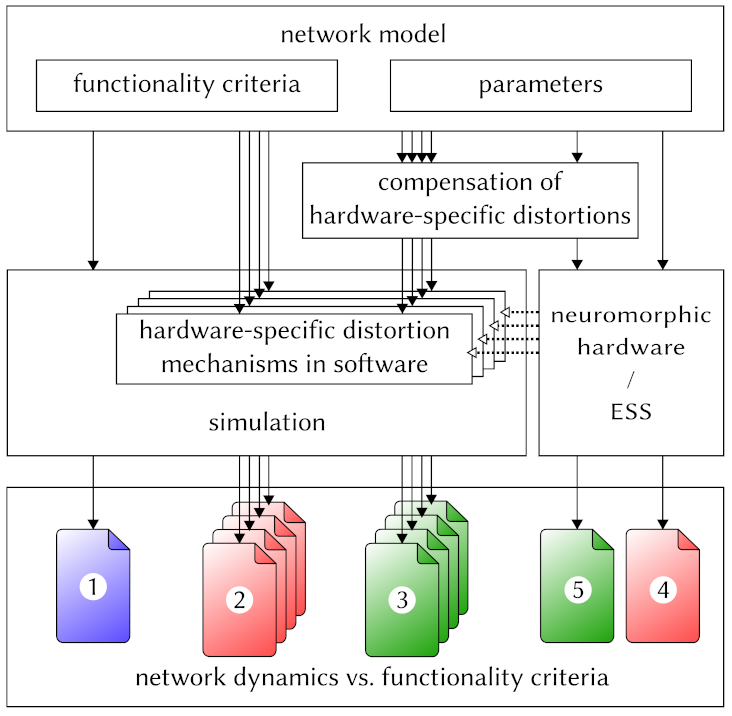
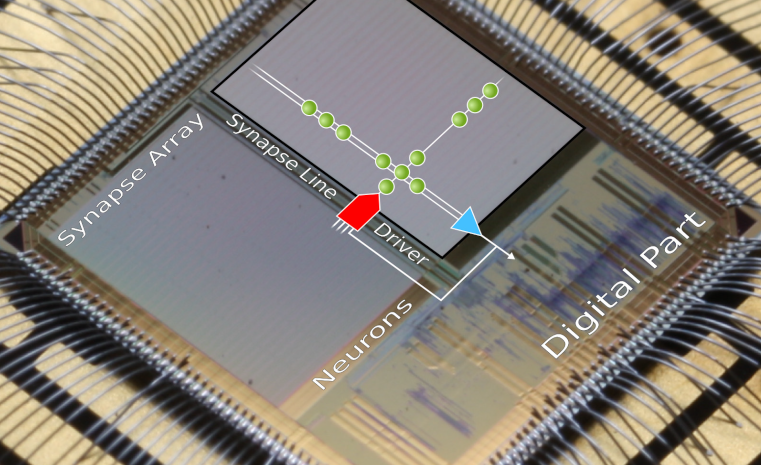
T. Pfeil, A. Grübl, S. Jeltsch, E. Müller, P. Müller, M.A. Petrovici, M. Schmuker, D. Brüderle, J. Schemmel, K. Meier.
Six networks on a universal neuromorphic computing substrate. Front. Neuroscience 2013 DOI
Six networks on a universal neuromorphic computing substrate. Front. Neuroscience 2013 DOI
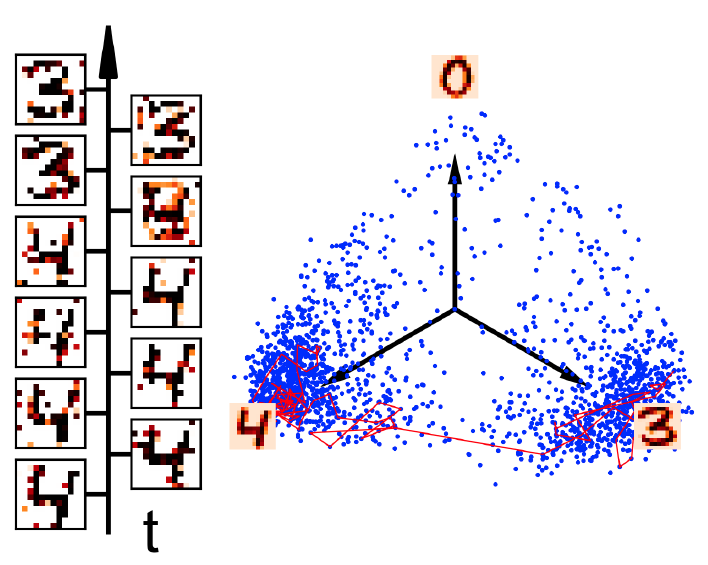
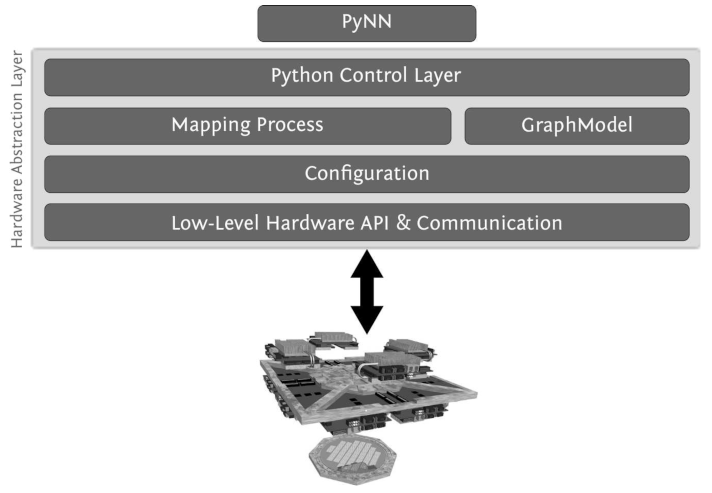
D. Brüderle, M.A. Petrovici, B. Vogginger, M. Ehrlich, T. Pfeil, S. Millner, A. Grübl, K. Wendt, E. Müller and M.O. Schwartz, K. Meier .
A comprehensive workflow for general-purpose neural modeling with highly configurable neuromorphic hardware systems. Biological Cybernetics 104, pages263–296, 2011 DOI
A comprehensive workflow for general-purpose neural modeling with highly configurable neuromorphic hardware systems. Biological Cybernetics 104, pages263–296, 2011 DOI
F Carminati, P. Foka, P. Giubellino, A. Morsch, G. Paic, J.-P. Revol, K. Safarík, Y. Schutz, A. Wiedemann, M.A. Petrovici, the ALICE Collaboration.
ALICE: Physics Performance Report, Volume I. J Phys G: Nucl. Part. Phys. 2004
ALICE: Physics Performance Report, Volume I. J Phys G: Nucl. Part. Phys. 2004