Neuro-inspired Theory, Modeling and Applications
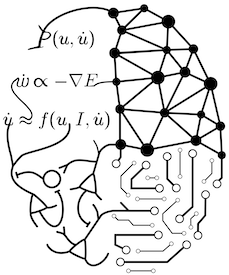
Our theoretical research is concerned with understanding and analytically describing various aspects of neural network dynamics. On the modeling side, we are mainly interested in functional networks (i.e., networks that do something that we consider useful), for which we take inspiration from both biology and AI research. An essential aspect of model functionality concerns robustness, since one of our goals is the embedding of functional networks in neuromorphic substrates and their application to real-world problems.
Our Research Interests
Highlights
Advancing AI with Brain-Inspired Computing: new contribution in ScienceNews!
Mihai A. Petrovici discussed the crucial role of neuromorphic hardware in the future of artificial intelligence and in enhancing AI efficiency and adaptability.
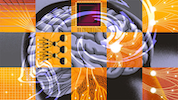
Read the article: More brainlike computers could change AI for the better
New NSF-SNSF Lead agency funded Project! - Start: 01.04.2025 / Runtime: 3 years
The NSF-SNSF Lead Agency process allows researchers in Switzerland and the US to submit a joint research proposal to either national funding agency.
The MINT project entitled "NSF-SNSF: Scalable accelerated learning in continuous neuronal systems" was awarded a total of $1,178,194.
$400,000 was awarded to the US collaborator Prof. Rajit Manohar and
to the project leader CHF 682,624 to the project leader Dr Mihai A. Petrovici.
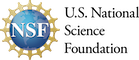
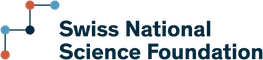
Lu.i: a palm-sized electronic neuron designed for educational and outreach purposes.
Lu.ineurons enable users to visualize and interact with the dynamics of individual neurons and small neural networks, effectively illustrating key neuroscience concepts. Its open-source and low-cost nature facilitates successful applications in classrooms and workshops.
For instance, the undergraduate students at the Technische Hochschule Nürnberg Georg Simon Ohm developed academic projects using the Lu.is neurons, which Prof. Axenie later showcased in his lecture on neuromorphic systems!
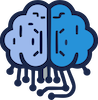
For more info, see academic neuromorphic projects:
Hybrid ArtificialNN and SpikingNN for closed-loop motor control and Spiking Silicon Neurons for Text2Morse Conversion
Forum für Universität und Gesellschaft - Nov. 02 - Info
The Forum provides free public access to expert knowledge to compile, clarify, and evaluate current information on selected topics. The 2024/25 series of event focuses on human insights in the era of artificial intelligence.
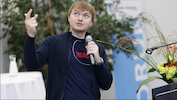
Watch Mihai A. Petrovici explaining how insights into brain learning mechanisms inspire the development of more efficient AI systems.
Das Gehirn als Vorbild: künstliche neuronale Netzwerke.
EBRAINS 2.0 - New funded Project! - Start: 01.01.2024 / Runtime: 3 years
The EBRAINS 2.0 project was submitted in response to the INFRASERV call (a Horizon Europe's Research Infrastructures actions) and was awarded €38 million to further develop the EBRAINS research infrastructure services, a legacy of the Human Brain Project.
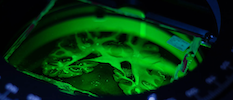
The project involves 59 partner institutions from 16 European countries and officially started in January 2024. As partners, our group together with CompNeuro were granted €1.1 million.