Dynamics and Statistics of Spiking Neural Networks
While it is quite apparent that any thermodynamic theory of neural networks must fall significantly short of explaining the vast repertoire of complex functionality exhibited by our brains, it remains highly instructive to understand how macroscopic observables can emerge from microscopic interactions between neurons.
Correlations in neural activity
When neural receptive fields become sufficiently large, shared-input correlations become inevitable in finite-size neural substrates. This induces correlations in their behavior, even if the two neurons have no direct synaptic connection. Such correlations affect a network's output statistics and thereby the information it computes and passes on. This is particularly relevant for neuromorphic devices, where communication is expensive in terms of chip area and external sources of activity must occupy the already limited bandwidth between the neuromorphic device and the host computer. Find out more: Petrovici 2016 - Bytschok 2011 - Bytschok 2017 - Dold et al. 2019 .
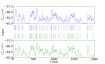
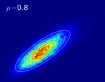
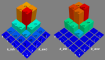
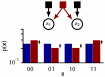
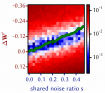
Asynchronous irregular spiking
Under appropriate parametrization, the self-sustained regime can constitute an attractor of a dynamical system. It is often observed that such an activity regime, characterized by low firing, weak correlations and highly irregular dynamics provides a good match to the dynamics observed experimentally in the awake, activated cortex. Thus, the ability to produce such asyncoronous irregular (AI) activity represents an interesting benchmark for neuromorphic hardware. One of the most intriguing questions regarding these firing patterns (or rather, lack thereof) is how they relate to computation. Find out more: Petrovici 2016 - Müller 2011 - Korcsak-Gorzo 2015 - Petrovici et al. 2014 .
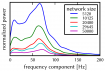
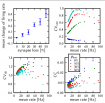
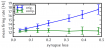
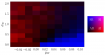
Neural sampling
In biological systems, randomness seems deeply embedded at all levels of neural information processing. Single neurons can therefore often be regarded as stochastic computing elements, with transfer functions that are shaped by some underlying noise-generating mechanism. Networks of such stochastic units can be thought of as performing a random walk in a very high-dimensional associated state space, i.e., sampling from an underlying (stationary) distribution. Understanding the origin and effect of noise on single neurons and neural ensembles is an important stepping stone in understanding biological neural dynamics and replicating them in artificial substrates. Find out more: Petrovici 2016 - Petrovici et al. 2016 - Petrovici et al. 2015 - Petrovici et al. 2015 - Jordan et al. 2019, - Dold et al. 2019 - Bytschok 2017 - Stöckel 2015 - Großkinsky 2016 - Kungl 2016 - Gürtler 2018 .
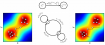
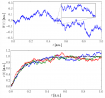
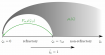
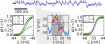
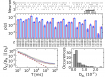
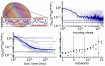
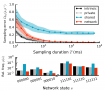
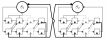
Ensemble phenomena
The identification of action potentials with state switches in binary spaces enables a straightforward connection to the behavior of magnetic systems. Many phenomena observed in solid state physics thus find their counterparts in the dynamics of spiking neural networks, such as hysteresis or phase transitions. However, due to their significantly more complex microscopic interactions, spiking networks exhibit new and interesting emergent phenomena. Find out more: Petrovici 2016 - Baumbach 2016 - Korcsak-Gorzo 2017 .
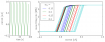
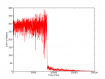
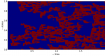
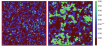
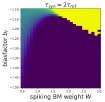
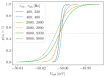